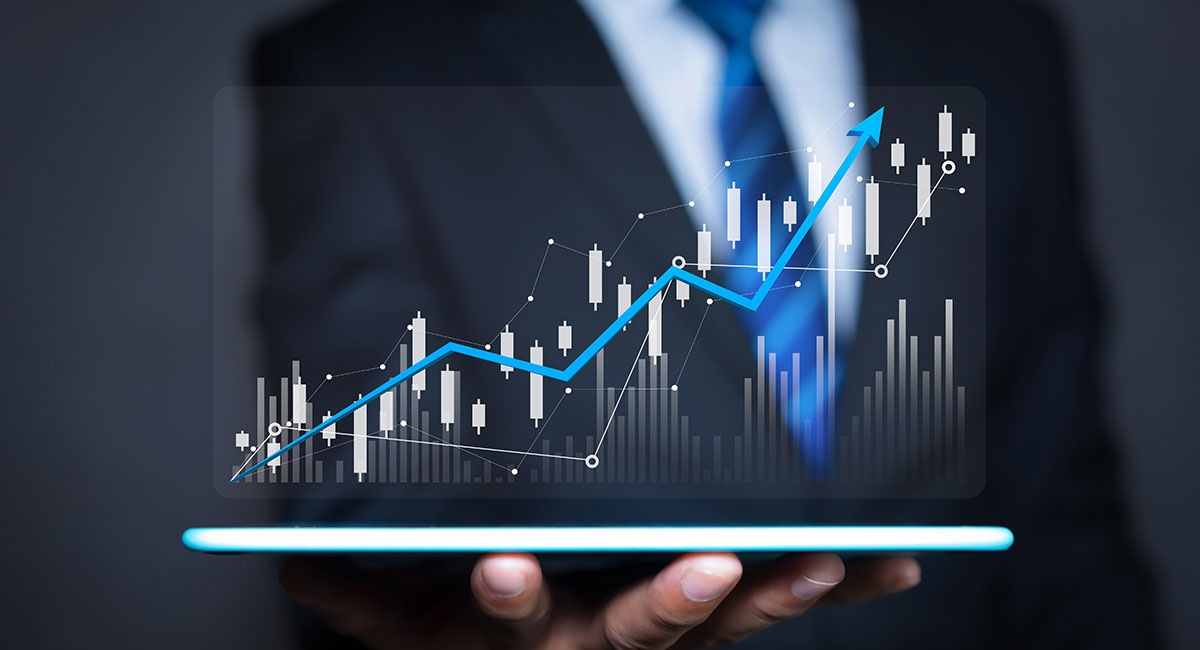
For MSPs monitoring thousands of IoT devices, parsing the associated data stream provides an outlet for growth. MSPs, however, must first acquire the essential skills.
Partners have an opportunity to expand their IoT services into the emerging field of analytics.
Indeed, CompTIA, an industry association, has identified three areas where IT service providers can engage in the IoT market: selling IoT devices, managing and monitoring them and data analytics. Providing data analytics in IoT, however, demands specialized skills.
“This takes analytics skill and training, as well as the ability to apply data findings to business opportunities, goals and challenges a customer might have,” said Carolyn April, senior director of industry analysis at CompTIA. Offering such a service “will require investment by channel firms in terms of human resources, technical and sales skills,” she noted.
The channel is “not quite there yet,” April added, but said data analytics is a focus area that has grown in importance across the last three years of CompTIA’s State of the Channel studies.
Early successes
Vivek Kaushik refers to IoT analytics as “IoT version 2.5,” explaining that the channel is still figuring out how to monetize the sale of IoT sensors and devices. But Kaushik, senior vice president of client services and account management at CSS Corp., a technology consulting services provider, called IoT analytics an “an exciting space.”
The company, based in San Jose, Calif., has started capturing data coming out of IoT devices “and we’re able to infer and create some predictive models to help [clients meet] the business challenge,” he said.
For example, CSS Corp. is working with one client to extract data from devices on farm tractors to predict a diagnostic issue and when a tractor would require service.
Similarly, the company is helping a locomotive components and operations client do predictive maintenance on railroad equipment to preempt device failure, optimize services and inventory, and update field engineers and procurement officials on when to order replacement parts.
In the railroad example, CSS Corp. has developed a six-step methodology that starts with identifying the most critical data associated with equipment for accurate predictions. Then the data is trained and tested in machine learning platform TensorFlow to build prediction models. Next, sensor data is merged with acoustics and image data to identify patterns, and then data from breaks, bearings and wheels is analyzed to identify wear and tear.
That way, CSS Corp. officials can identify uncharacteristic system behavior and resolve issues before they occur, as well as identify the best maintenance schedule and make recommendations to clients to optimize processes.
The next step is to see if the firm can bring in another layer of intelligence to see why some equipment performs better than others, Kaushik said. Beyond analyzing historical data to predict a maintenance failure, now CSS Corp. officials want to see if they can use a locomotive company’s sensors and IoT data to suggest whether a different maintenance process would extend the life of a piece of equipment such as a wheelbase.
This takes analytics to the augmented level, he said. Augmented analytics includes information coming into a centralized platform from different sources, including a locomotive company’s competitors, or other trains in the same company. “Maybe they’re oiling [the wheelbase] two times a day and they need to do it three times a day,” he said. The idea is to take best practices from what other trains are doing and moving from predictive to suggestive, which is the augmented component.
AI will play a big role in making this possible because a system becomes increasingly intelligent by consuming more data and doing more repetitive tasks, Kaushik said. “This is the journey we’ll see more and more.”